Predictive Analytics: Using AI to Forecast Student Performance and Outcomes
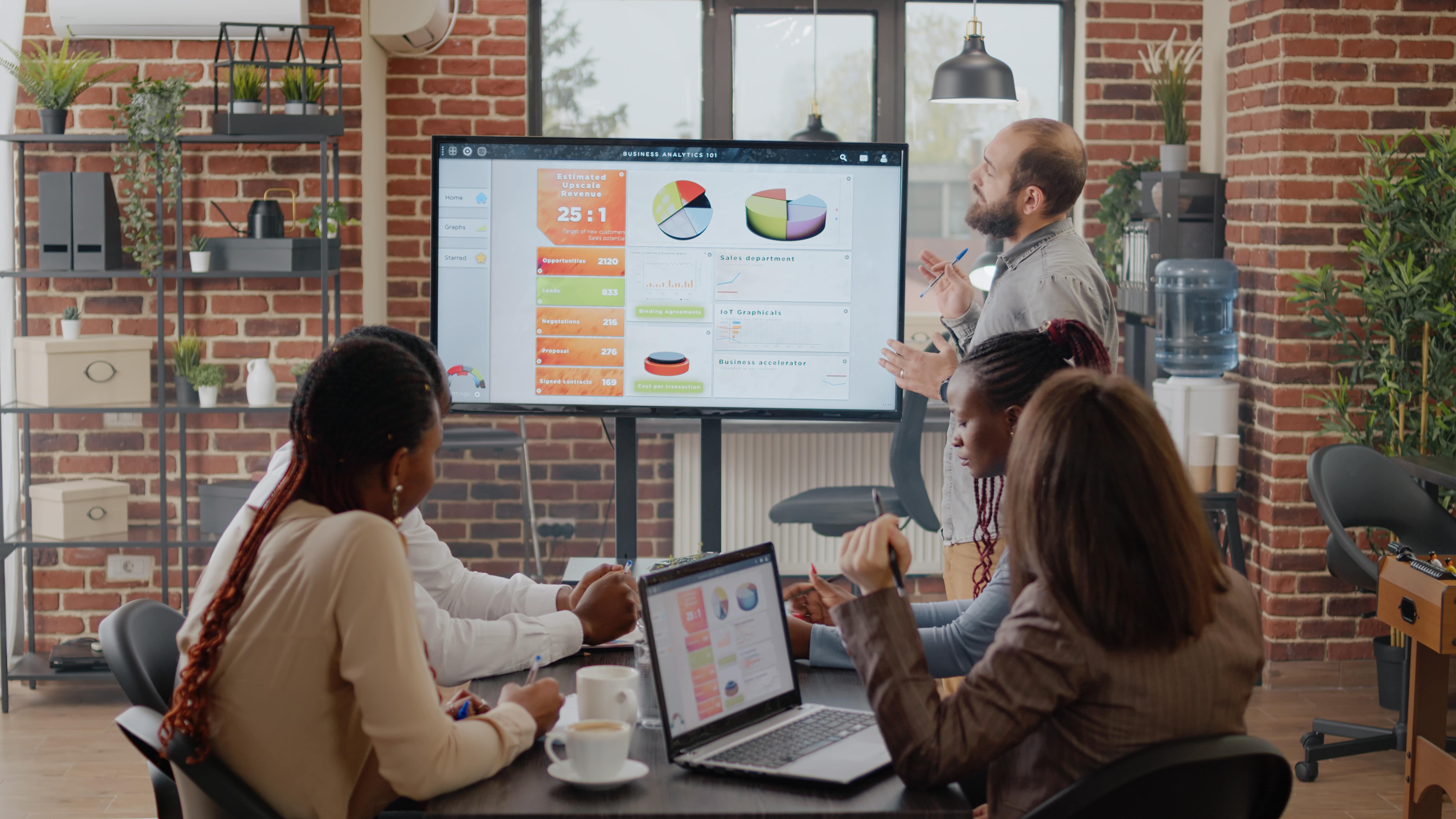
In recent years, educational institutions have increasingly turned to advanced technologies such as artificial intelligence (AI) to enhance learning outcomes and operational efficiency. One of the most powerful applications of AI in education is predictive analytics, a method that uses historical and real-time data to forecast future events. In the academic context, predictive analytics can anticipate student performance and outcomes, enabling early interventions, tailored support, and improved educational planning.
What is Predictive Analytics?
Predictive analytics involves using data mining, statistical algorithms, machine learning, and AI techniques to identify patterns in data and predict future trends and behaviors. When applied to education, this method can provide insights into various aspects of student performance, such as grades, dropout rates, and the likelihood of completing a degree. The core idea is that by analyzing historical data — including attendance records, prior academic achievements, extracurricular activities, and even social media engagement —educational institutions can forecast which students might need extra help or which strategies are most effective for enhancing learning.
The Growing Role of AI in Education
AI in education is proliferating. According to Research and Markets, the global AI in education market is projected to reach $20 billion by 2027, growing at a compound annual growth rate (CAGR) of 45%. Predictive analytics is a significant part of this surge, helping schools and universities streamline processes, optimize learning outcomes, and personalize the educational experience.
In particular, AI-driven predictive models can transform educational systems in several key ways, such as:
- Early identification of at-risk students: By identifying students likely to struggle academically, schools can intervene early, providing targeted support that can help improve retention rates and overall student success.
- Personalized learning pathways: AI can recommend customized learning plans based on student strengths, weaknesses, and interests, ensuring that education is tailored to individual needs.
- Efficient resource allocation: Predictive analytics helps schools allocate resources such as tutoring services or mental health support more efficiently by identifying which students will benefit most.
The Mechanisms of Predictive Analytics in Education
AI and machine learning algorithms process various data points to predict student performance. Here are some of the most important types of data used:
- Academic Performance Data: This includes grades, standardized test scores, course completion rates, and other measures of student academic success. These data points provide insight into student learning patterns and areas where intervention might be needed.
- Behavioral Data: Attendance records, participation in classroom activities, homework completion rates, and extracurricular involvement are valuable indicators of student engagement and commitment to learning.
- Social and Demographic Data: Family background, socioeconomic status, and parental education can influence student outcomes. While these factors are often outside the school’s control, they help identify trends and patterns that might impact performance.
- Learning Management System (LMS) Data: Online learning platforms collect vast amounts of data about student activity, including how often they log in, which resources they access, and how they interact with digital content. This data provides real-time insight into student learning habits.
The Impact of Predictive Analytics on Student Outcomes
The ability to predict student outcomes can profoundly impact both short-term academic performance and long-term career success. Some key benefits include:
- Increased Retention and Graduation Rates: Predictive analytics can help address this by identifying students who are at risk of dropping out. By understanding the factors contributing to attrition, schools can provide more targeted support, such as academic advising, counselling services, or financial aid.
- Improved Student Engagement: Predictive models can identify patterns in student behavior that signal disengagement, such as skipping classes or falling behind on assignments. With this information, educators can intervene early to re-engage students, whether through tutoring, personalized feedback, or adjustments to the curriculum.
- Personalized Learning: One of the most exciting predictive analytics applications is its ability to deliver personalized learning experiences. By analyzing a student’s strengths, weaknesses, learning style, and progress, AI can suggest customized learning pathways, recommend supplementary materials, or even adjust the pacing of lessons to suit the student’s needs.
- Data-driven Decision-making: Predictive analytics allows administrators to make more informed decisions about curriculum design, resource allocation, and policy changes. For example, if the data shows that specific courses have high failure rates, administrators can investigate whether changes in teaching methods, additional support, or adjustments to course materials are needed.
Ethical Considerations and Challenges
While predictive analytics offers many benefits, it raises significant ethical concerns regarding privacy and data security. Schools must ensure that they are handling student data responsibly and transparently. There is also the risk of over-reliance on algorithms, which can reinforce biases or make incorrect predictions. For example, if a predictive model is based on historical data that reflects systemic inequalities, it could unfairly categorize students from specific backgrounds at higher risk of failure.
Another challenge is ensuring that predictive analytics tools support, rather than replace, educators’ roles. AI can provide valuable insights, but it should be seen as a complement to the human element of teaching, not a substitute for it.
Future Directions
The future of predictive analytics in education is promising. With more sophisticated AI models and the increasing availability of real-time data, predictive analytics will become even more accurate and actionable. Some of the emerging trends include:
- Real-time interventions: AI systems can provide instant feedback and intervention based on real-time data, helping students stay on track in the moment rather than after problems have escalated.
- Integration with other emerging technologies: Predictive analytics will likely be combined with different technologies, such as virtual reality (VR) and augmented reality (AR), to create immersive learning experiences tailored to individual needs.
- Expansion to K-12 education: While much of the current focus is on higher education, K-12 schools are also beginning to adopt predictive analytics to improve student outcomes, particularly in early literacy and math skills.
Conclusion
Predictive analytics transforms the education landscape by providing valuable insights into student performance and outcomes. Using AI and machine learning, schools and universities can identify at-risk students, tailor learning experiences, and improve overall academic success. However, as with any technology, it must be used responsibly, carefully considering ethical implications. As predictive analytics continues to evolve, its potential to revolutionize education will only grow, offering new opportunities to enhance student learning and success.
Also, read these blogs to get more insights on Proctoring:
- 7 Ways to Create a Stress-Free Remote Proctoring Experience for Students
- The Accessibility of Remote Proctoring for Students with Disabilities