NLP in Assessments – Revolutionizing Education and Evaluation
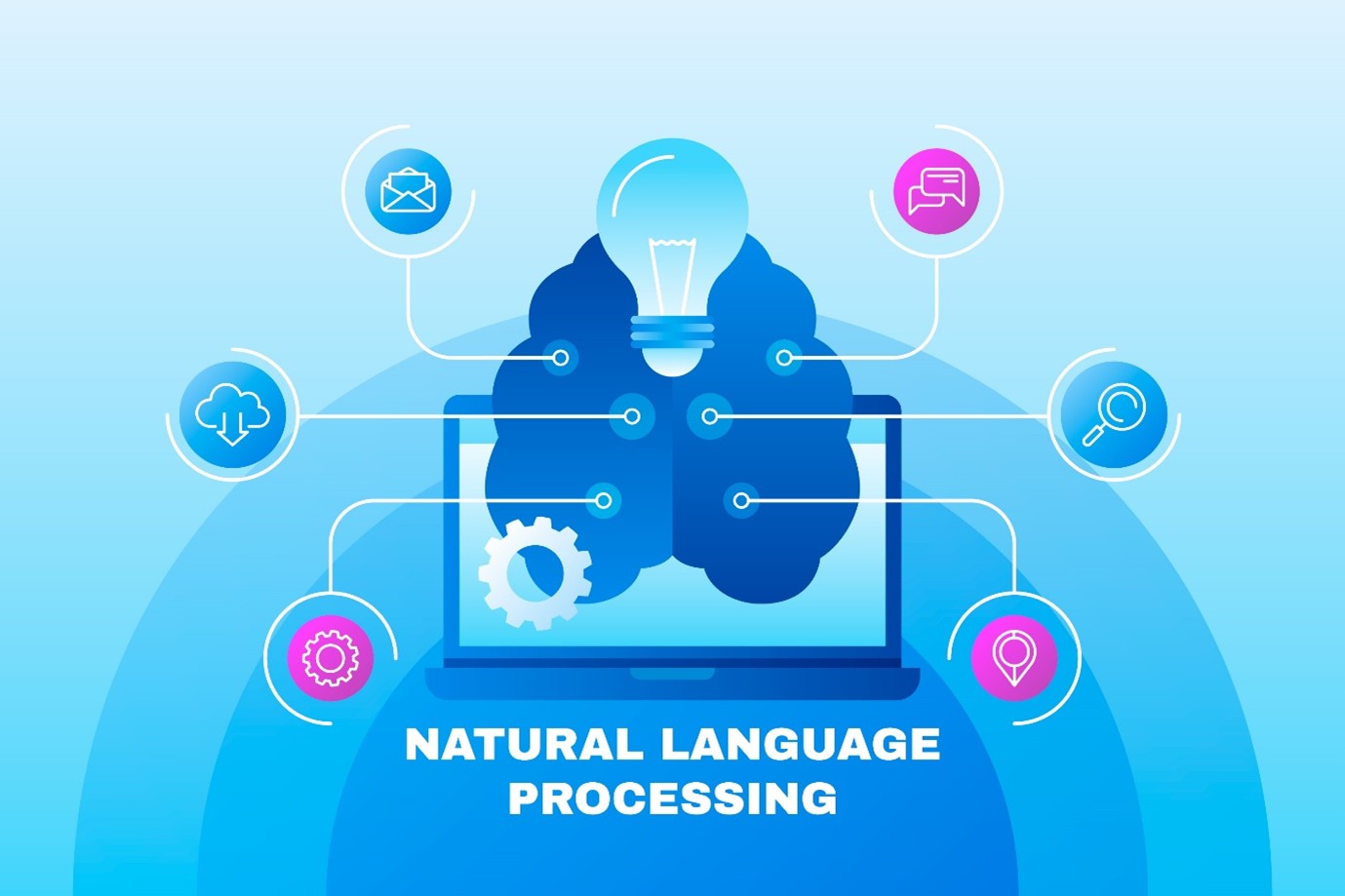
Introduction
Natural Language Processing (NLP), a subfield of artificial intelligence (AI), has become integral to modern technology. It enables computers to understand, interpret, and generate human language; NLP powers many daily tools, from virtual assistants to machine translation. In education, NLP is increasingly used to revolutionize assessments, making the evaluation process more efficient, personalized, and data-driven. By analyzing language in student responses, NLP can offer insights into learning patterns, provide real-time feedback, and reduce the workload of educators, all while maintaining fairness and consistency.
This article explores how NLP is transforming assessments in education, including the benefits and challenges, focusing on its application in essay grading, personalized feedback, and formative assessments.
The Role of NLP in Education
In the digital age, education is evolving to meet the needs of both students and educators. With growing class sizes and increasing diversity in student needs, traditional methods of assessment — particularly essay grading and feedback — have struggled to keep pace. NLP offers solutions that help streamline this process, such as:
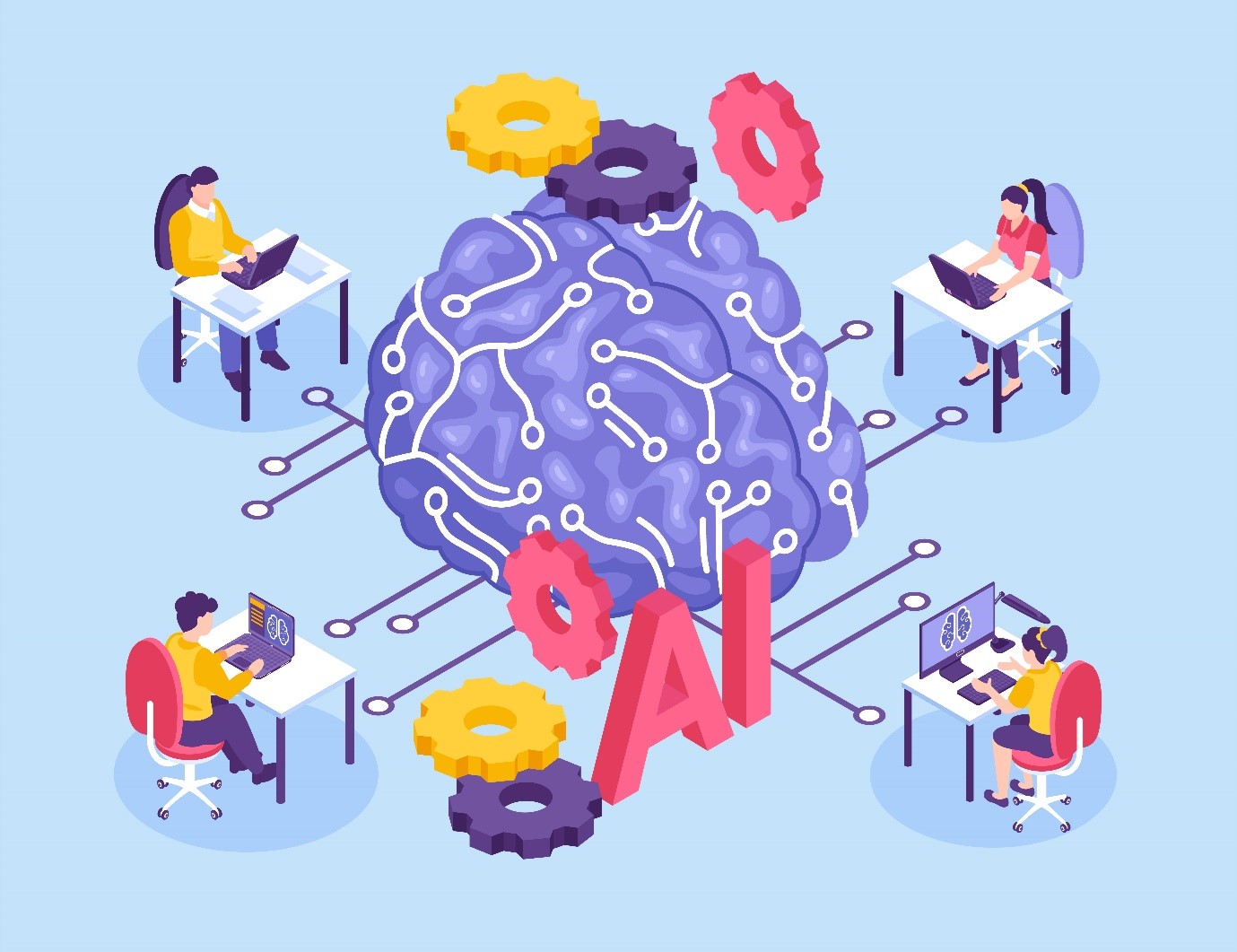
Automated Essay Scoring (AES)
One of the most prominent applications of NLP in education is Automated Essay Scoring (AES), which uses AI-powered systems to assess and grade student essays. Traditional grading methods are often time-consuming and subject to human bias. AES systems address these issues by evaluating essays based on predefined criteria such as grammar, coherence, relevance, and depth of content.
The earliest AES systems were primarily rule-based, relying on statistical models to assess writing quality. However, advancements in NLP and machine learning have significantly improved the accuracy and efficiency of these systems. Today’s AES tools can understand the context, detect semantic nuances, and evaluate the overall coherence of an essay. In addition to standardized tests like the GRE, AES systems are used in K-12 education and MOOCs (Massive Open Online Courses). With NLP, assessments can be scaled to hundreds or even thousands of students without sacrificing quality, ensuring timely and relevant feedback.
Real-Time Feedback and Adaptive Learning
Beyond just grading, NLP has made significant strides in providing real-time feedback to students. In formative assessments, immediate feedback is essential for reinforcing learning. NLP-powered tools can analyze student responses, identify mistakes, and provide corrective feedback instantly, allowing students to learn from their errors before moving forward. Real-time feedback benefits students in online courses or self-paced learning environments where instructors may not be immediately available to provide support.
For example, tools like Grammarly and Turnitin’s Feedback Studio use NLP algorithms to analyze text, detecting grammar mistakes, stylistic issues, and even plagiarism. They can suggest alternative phrasings, point out common errors, and explain the corrections, which can help students develop better writing skills over time.
In addition, NLP can power adaptive learning platforms, which use real-time data to adjust the difficulty and content of assessments based on the student’s progress. These platforms use NLP to interpret student responses, predict learning patterns, and personalize the learning experience, ensuring that students receive the right level of challenge at the right time.
Enhancing Language Assessments
NLP is also making its mark in language assessments, particularly second-language acquisition (SLA). Traditional language assessments rely on human examiners to evaluate students’ speaking, listening, reading, and writing proficiency. NLP can streamline this process by automating the evaluation of spoken and written responses.
For instance, the Duolingo English Test uses NLP to assess test-takers’ English proficiency in real time. The test uses both speech recognition and text analysis algorithms to evaluate speaking fluency, grammar, and vocabulary usage.
Bias Reduction and Fairness
One of the critical challenges in human-based assessments is the potential for bias. Handwriting quality, gender, race, or unconscious preferences can influence grading outcomes. When designed carefully, NLP systems can help mitigate these biases by adhering to consistent evaluation criteria.
However, it is essential to note that while NLP can reduce certain biases, it is not entirely free from them. If trained on biased data, NLP models can perpetuate existing inequalities. For instance, an AES system trained predominantly on essays from native English speakers might struggle to assess non-native speakers fairly, penalizing them for unconventional syntax or idiomatic usage.
To address these concerns, developers are improving NLP models’ fairness and transparency. For instance, open-source datasets that include diverse linguistic styles, dialects, and cultural contexts are used to train more equitable models. Researchers are also exploring Explainable AI (XAI) techniques, which aim to make the decision-making processes of AI models more transparent and understandable to educators and students.
Challenges and Limitations of NLP in Assessments
While NLP has shown tremendous potential in transforming educational assessments, it has challenges. Some of the challenges include:
- Contextual Understanding: Although modern NLP models are adept at recognizing grammatical structures and surface-level content, they still need help with deeper semantic understanding, particularly in more abstract or creative responses. For instance, evaluating essays requiring critical thinking or analyzing poetry remains challenging for NLP systems.
- Training Data: NLP models require vast amounts of high-quality data to function effectively. Acquiring sufficient training data representing diverse student populations can be complex in many educational settings.
- Ethical Concerns: Privacy concerns also arise when using NLP for assessments. Educational institutions must ensure student data is handled responsibly and complies with privacy regulations.
- Over-reliance on AI: There is a risk of over-reliance on automated systems, potentially diminishing the role of human educators. While NLP can assist in providing scalable and timely assessments, the importance of human judgment, particularly in complex learning environments, can only partially be replaced.
Future of NLP in Assessments
The future of NLP in education looks promising. As algorithms become more sophisticated and data collection methods improve, NLP models will be better equipped to handle nuanced student responses and provide more personalized feedback.
Moreover, integrating NLP with other AI technologies will likely result in even more robust and holistic assessments of student learning.
Conclusion
Natural Language Processing is transforming the educational landscape by enabling more efficient, scalable, and personalized assessments. From automated essay scoring to real-time feedback and language assessments, NLP is helping educators evaluate student performance more effectively while reducing bias and improving fairness. However, ongoing research and ethical considerations will be critical to fully harnessing the potential of NLP in assessments. As the field continues to evolve, NLP has the potential to redefine how we measure and support student learning in a data-driven and equitable manner.